外文翻譯-二級斜齒圓柱齒輪減速器自動優(yōu)化設(shè)計
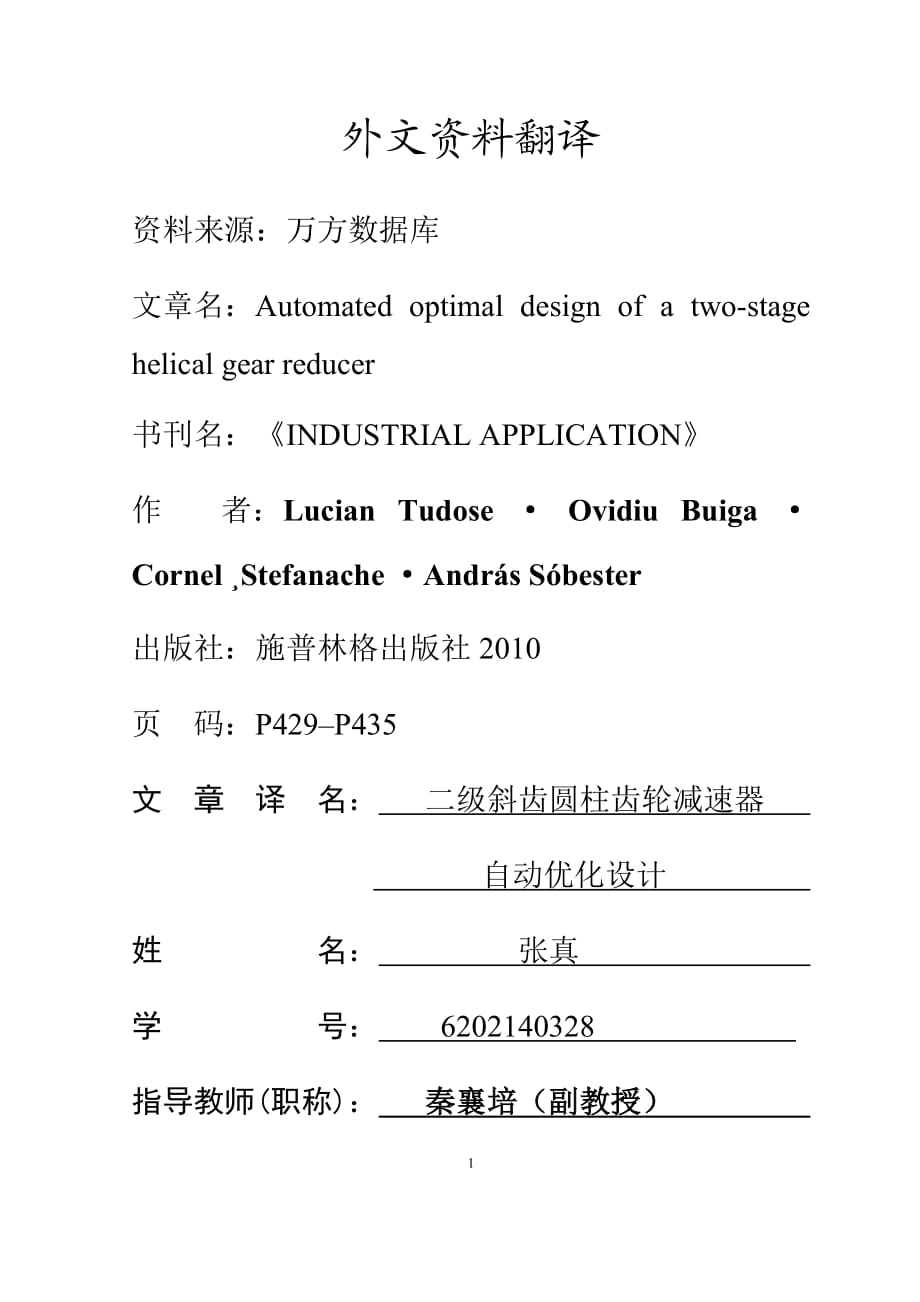


《外文翻譯-二級斜齒圓柱齒輪減速器自動優(yōu)化設(shè)計》由會員分享,可在線閱讀,更多相關(guān)《外文翻譯-二級斜齒圓柱齒輪減速器自動優(yōu)化設(shè)計(24頁珍藏版)》請在裝配圖網(wǎng)上搜索。
1、 外文資料翻譯 資料來源:萬方數(shù)據(jù)庫 文章名:Automated optimal design of a two-stage helical gear reducer 書刊名:《INDUSTRIAL APPLICATION》 作 者:Lucian Tudose Ovidiu Buiga Cornel Stefanache Andrs Sbester 出版社:施普林格出版社2010 頁 碼:P429–P435 文 章 譯 名: 二級斜齒圓柱齒輪減速器 自動優(yōu)化設(shè)計 姓 名: 張真
2、 學(xué) 號: 6202140328 指導(dǎo)教師(職稱): 秦襄培(副教授) 專 業(yè): 機械設(shè)計制造及其自動化 班 級: 12機制03 所 在 學(xué) 院: 機電學(xué)院 英文文獻: Automated optimal design of a two-stage helic
3、al gear reducer Lucian Tudose Ovidiu Buiga Cornel Stefanache Andrs Sbester Received: 28 August 2009 / Revised: 21 February 2010 / Accepted: 6 March 2010 / Published online: 28 March 2010_c Springer-Verlag 2010 Abstract The design space of multi-stage transmissions is usually very large
4、and heavily constrained. This places significant demands on the algorithm employed to search it,but successful optimization has the potential to yield considerably better designs than conventional heuristics, at the same time enabling a better understanding of the trade-offs between various obje
5、ctives (such as service life and overall weight). Here we tackle a two-stage helical gear transmission design problem (complete with the sizing and selection of shafts, bearings, housing, etc.) using a two-phase evolutionary algorithm in a formulation that can be extended to include additional stage
6、s or different layouts. Keywords Evolutionary optimization Gear train design Spur gear sets Punctuated equilibria Multi-objective optimization 1 Introduction The complexity of the design of multi-stage reducers lies in the strong and often intractable connections between the design vari
7、ables defining its sub-systems. In other words, an optimal reducer is generally not an assembly of components optimized in isolation, a fact overlooked by many conventional design heuristics. For instance, the impact of a certain choice of gear width and center distance may yield a minimum mass gear
8、ing, but the selection of this gearing may cascade through subsequent steps of the design process (sizing of shafts, further stages, bearings, housing, etc.) to ultimately lead to a heavier reducer than if a slight compromise had been made on the choice of that first gearing. A typical example migh
9、t be that selecting a smaller than optimal gear diameter (and a correspondingly greater contact width) could yield a somewhat heavier gearing,but a more compact layout and therefore a much lighter housing; it is worth mentioning though that in reality the impact on the overall objective tends to be
10、much less direct and therefore much more obscure than in this example. Of course, in all but a few trivial cases, it is impossible to tell what that first compromise should have been, let alone what any subsequent choices should have been made with the overall goal in mind, instead of concentrating
11、 on the subsystem in hand. The chief reasons impeding a truly ‘holistic’ reasoning at every step of the design heuristic are the sheer number and the highly non-linear nature of the constraints and the objectives, the large number of design variables and the complexity of the interactions between th
12、em. Additionally,analytical models may not be available for these interactions and constraints, precluding higher level analytical calculations that could predict the global effect of local design decisions. The last two decades have seen an increasing awareness amongst the power transmission desig
13、n community of the shortfalls of simple trial and error type methods conventionally used to tackle this highly constrained class of design problems and potential replacements have begun to emerge in the shape of expert systems (Ferguson et al.1999; Abersek et al. 1996), synthesis tools based on spat
14、ial grammars (see the simulated annealing-driven, grammar based topological gearbox design tool described by Lin et al. 2009), particle swarm searches (Ray and Saini 2001),algorithms based on the modeling of civilizations and societies(Ray and Liew 2003), constrained quasi-Newton local searches (see
15、 the study by Thompson et al. 2000) into the fatigue life versus gearing volume trade-off) and evolutionary algorithms (the work of Li et al. (2008) on the application of a fuzzy-controlled genetic search to the optimization of a simple reducer model and the study by Gologlu and Zeyveli (2009) for r
16、ecent examples). In fact, the latter category—headlined by genetic algorithms (GAs)—appears to be the direction of choice at present and there are two key reasons for this. Firstly, GAs can handle the highly discretised design spaces of transmission systems. Standardisation and the favouring of of
17、f-the-shelf (as opposed to purpose-designed) subcomponents are the main reasons for most design variables only being permitted a pre-determined set of discrete values (as we shall see, this is the case with our own application too). Secondly, the full description of a class of reducers (say, that of
18、 the two-stage, helical gear family) generally requires a large number of design variables—typically, well over ten—and GAs have a fine track record in the global search of very large design spaces, especially when the computation of the goal function and the constraints is comparatively inexpensiv
19、e. The motivation behind the work described here is that evolutionary computing technology has now reached the level where, we believe, it is computationally feasible to consider the automated optimal design of complete reducers.The experiments referenced above have been instrumental in highlighti
20、ng the importance of using modern global optimization techniques in transmission design (as opposed to conventional, trial and error type design algorithms) even when considering certain subproblems—here we propose to extend the technology to the broader design space of a two stage reducer, whose e
21、very element (bearings, seals, shafts, etc.) is subject to change throughout the optimization process. The industrial relevance of the exercise is ensured by the consideration of all design constraints typically encountered in practice—we bound the design space by a total of 77 constraints categori
22、zed into 24 groups. In Sections 3 and 4 we discuss this formulation in detail, with Section 5 containing an account of the results of its deployment. First, however, we need to introduce a key element of our evolutionary computingmethodology, necessitated by the need to handle such a large number of
23、 constraints in an efficient manner. 2 An evolutionary paradigm The inevitable paucity of the fossil record makes it rather difficult to estimate the rate of evolutionary change along any given lineage. Nevertheless, it is almost certain that evolution proceeds with varying speed.The extrema o
24、f these speed variations are, however, subject to some debate in the evolutionary biology community. It is clear from neo-Darwinian synthesis that large changes (macromutations) are almost always deleterious and this is an evolutionary upper speed limiting factor. According to the school of thought
25、usually associated with the seminal paper of Eldredge and Gould (1972), the lower limit is practically zero. That is, they suggest that populations evolve in bursts,which punctuate long periods of equilibrium (stasis), when no variations occur. From the perspective of evolutionary algorithm design
26、it is almost entirely irrelevant whether the theory of punctuated equilibria is correct or not. After all, GAs incorporating spells of Lamarckian learning are not made less successful by the fact that the Lamarckian theory of evolution is now known to be incorrect! Similarly, macromutations are ofte
27、n beneficial in evolutionary optimization, reminding practitioners that evolutionary computation is not an exact simulation of nature (nor was it meant to be). Consequently,it is not surprising that the idea of punctuated equilibria has seen steady exposure over the years in the evolutionary algorit
28、hms community, in spite of the debate surrounding it in biology. This exposure is associated with two main lines of intellectual inquiry. Firstly, many practitioners of evolutionary optimization have noted periods of stasis on multi-modal fitness landscapes.The familiar pattern sees the population
29、converging on a local optimum, where a number of generations of stagnation (metastability) precede a beneficial mutation, which propels the population into the next, better basin of attraction (see, for example, Oh and Yoon 1998 for a detailed account of the dynamics of this phenomenon on a one-dime
30、nsional bistable fitness landscape). Secondly, and this is the angle we are interested in here,some success has been reported over the years in attempts to actually engineer metastable states in GAs, which would then be followed by bursts of rapid convergence. There is no unique, well-defined templ
31、ate for designing such heuristics; the literature contains a fairly broad range of models that are loosely based on the concept of punctuated equilibria. Much of the work along these lines is based on multipopulation architectures. An example is the integrated circuit design application of Cohoon e
32、t al. (1991), where each subpopulation (environment) is allowed to evolve independently for a number of generations (an epoch), after which a ‘natural catastrophe’ causes genetic material to move between subpopulations, followed by another epoch of isolated evolution, etc. Multiple epochs separated
33、by catastrophes can be seen in single population implementations too—see, as an example, the GA of Hamada et al. (2001), where the catastrophe is represented by a steep increase in mutation rates. Indeed, a single-population, multi-epoch framework defines the structure of our proposed search strat
34、egy too. We depart, however, from the previously mentioned heuristics in the way we control the evolution of the population within each of two distinct types of epoch (or phase). In the first, the population is only subjected to the selective (evolutionary) pressure of the constraints. The epoch con
35、cludes when a sufficient number of feasible individuals has been generated—this is one of the run-time parameters of the heuristic. Typically this threshold value is set to around 40% of the population—clearly, this value controls the balance between feasibility and diversity. The next epoch then se
36、es most of the control of the selective pressure relinquished to the objective function itself; at this stage, the algorithm works like a standard GA, which penalises constraint violations in the conventional manner. Upon registering a drop in the feasibility percentage of the population below a cer
37、tain threshold value, the algorithm reverts once more to the constraint-led mode of operation for the next epoch and so it proceeds until some termination condition is met (typically, the objective value of the fittest individual reaches a pre-set threshold). As noted before, this novel constraint-
38、handling mechanism is the result of our efforts to solve a problem subject to a very high number of constraints. We shall review the constraints of our reducer design problem shortly, but first let us consider the ‘genotype’, or the set of design variables, that defines the two-stage reducer. 3
39、 The ‘genotype’ of the two-stage reducer The class of reducers we are considering here (see Fig. 1 for an example) is highly standardised, both in terms of the design of their gearings and bearings and in terms of their layout. The set of 18 design variables that define the reducer unequivocally (s
40、ee Table 1) reflects this, with standardisation imposing discrete value sets on most of them. Nevertheless, the resulting design space is still vast. Assuming a discretisation of the few continuous variables into 25 steps, we could obtain a number of possible reducer designs of the order 4 1026 (i
41、t is notoriously hard to gain an intuitive ‘feel’ for such numbers, but it is worth considering that this is comparable to the number of atoms in about eight kilograms of C-12!). Two conclusions can be drawn from here. Firstly, it is clear that, although the computational cost of evaluating the obj
42、ective function and the constraints for a given design is Fig. 1 Sketch of the two-stage reducer quite low (less than a second per design), an exhaustive (full factorial) search of the design space is not feasible. Secondly, of all the search heuristics one could consider using instead, population-
43、based evolutionary algorithms appear to be the most suitable, reinforcing the observation we made earlier regarding the popularity of such approaches in the transmission design literature. 4 A highly constrained design space This is probably a good time to turn our attention to the constraints
44、 of our design problem, which, as hinted earlier, constitute the key challenge of this class of problems in general and of optimizing the structural elements of the reducer shown in Fig. 1 in particular. As it will become apparent, they are all of the inequality type, mostly involving geometrical or
45、 structural considerations. There are a total of 77 constraints, which we have organized into 24 groups (e.g., the same type of constraint applied to all four gears constitutes one group, though it is actually implemented as four separate constraints). In the interest of conciseness we shall not d
46、well on the details of their calculation (the interested reader may find all the details of the gearing calculations in the relevant industrial standard document (DIN 1987) and the catalogs and calculation methods used for bearings and seals in the SKF online catalog ( Accessed on 17 August 2009));
47、we do note though that they are mostly based on a combination of lookup tables and simple analytical models, their computational cost therefore being minimal. Additionally, it is worth noting that to enable the calculation of some of the constraints (such as some of the geometrical inequalities, a
48、s well as those related to lubrication and operating temperature), the housing was also designed automatically once the virtual design of each two-stage gearing was generated. The following list of constraints should be viewed with reference to the sketch in Fig. 1. Constraint group 1 The relati
49、ve dif ference between the required and the actual gearing ratio must be within the range [?2.5%, 2.5%] on both stages. Constraint group 2 The Hertzian contact pressure on the teeth of both stages must not exceed a specif ied value. Constraint group 3 The bending stress on the teeth of gears 1
50、 through 4 must not exceed a specif ied value. Constraint group 4 The teeth on gears 1 through 4 must not be undercut. Constraint group 5 The top land of the teeth on gears 1 through 4 must not vanish. Constraint group 6 The contact ratio on stages I and II must be greater than a specif ied va
51、lue. Constraint group 7 The normal addendum coef f icients on both stages should be in the range [?0.5, 1]. Constraint group 8 Measurability constraint for all four gears. Constraint group 9 The numbers of teeth on the gears of both stages must be relative primes. Constraint group 10 Gear 2 m
52、ust not interfere with the output shaft. Constraint group 11 Lubrication constraint—the margin between the minimum and maximum allowable lubricant levels should be no less than 10 mm. Constraint group 12 The input and output shaft ends must have a suf f icient diameter step to allow the mounti
53、ng of a belt wheel. Constraint group 13 The inside diameter of the tapered roller bearings on the input and output shafts must be less than the mounting diameter of the seal. Constraint group 14 Geometrical constraint relating to the space required by the outside ring of the tapered roller be
54、arings on the input and output shafts. Constraint group 15 Set of manufacturability constraints on all four gears. Constraint group 16 Input, output and intermediary shaft stress constraints (radial and axial loads originating from the gearings). Constraint group 17 The fatigue life safety fac
55、tors on the three shafts must not fall below a specif ied value. Constraint group 18 The bending strains on the three shafts in key locations must be below certain threshold values to enable the correct functioning of the gearings and the bearings. Constraint group 19 The torsional strains in
56、the three shafts must be below a threshold value. Constraint group 20 The service life of the tapered roller bearings must exceed a specif ied value. Constraint group 21 The shearing and crushing stresses must not exceed a specif ied value on the keys and keyways of the input and output shafts
57、. Constraint group 22 The minimum distance between adjacent roller bearings must be greater than 15 mm. Constraint group 23 The shearing and crushing stresses must not exceed a specif ied value on the keys and keyways used the attach the four gears to the shafts. Constraint group 24 The operat
58、ing temperature of the reducer must not exceed a specif ied value. 5 A mass minimization problem Let us now consider the following design problem. A 2.9 kW two-stage reducer is to be designed for minimum weight and a service life of 8,000 h, given an input speed of 925 RPM and a transmission
59、ratio of 7.6. The gears should be based on an ISO 53 basic rack profile, with the pinions and wheels made of quenched and tempered 42CrMo4 and 41Cr4 respectively. Running the algorithm described earlier yielded a reducer with a 2.8 2.7 division of the transmission ratio and axial distances of 80 a
60、nd 100 mm on stages one and two respectively, weighing 44.3 kg. This solution was found on the boundary of the second transmission stage Hertzian contact pressure constraint, very near to four additional constraint boundaries. These are highlighted in Fig. 2. In contrast to the successful determina
61、tion of this global optimum found in an ‘a(chǎn)wkward’ corner defined by several constraint boundaries, consider the outcomes of a set of benchmark experiments. The natural basis for comparison is, of course, the standard GA the multi-epoch heuristic is built upon, run with the epoch switching feature di
62、sabled. Multiple repeated runs of this GA failed to reach the objective value of 44.3 kg within a budget of 300,000 evaluations. In fact, this standard GA failed to reach even a slightly relaxed threshold objective value of 45.8 kg by an arbitrarily selected cut-off point of 300,000 evaluations. By
63、comparison, the multi-epoch algorithm has attained this value on every one of 50 independent runs (started from different random initial populations) of up to 300,000 evaluations— Fig. 3 is a histogram of the number of evaluations required by it to do so (average of just under 75,000 evaluations, st
64、andard deviation of just over 54,000). Perhaps even more tellingly, as the first bar of the histogram shows, 17 of the 50 runs passed the threshold weight value after less than 50,000 evaluations. As an additional benchmark, we have also tested a Simulated Annealing optimizer (an implementation of
65、 a recent version of the heuristic by Talbi 2009) as a means of tackling the reducer problem. Experiments with five different types of cooling schedules (linear, exponential, parabolic, hyperbolic and power) run to 1,000,000 evaluations of the objective function, failed, just as in the case of the “
66、plain” Genetic Algorithm, to reach the threshold weight, once again underscoring the extreme difficulty of this mixed variable problem. In addition to the solution of the basic design problem, the type of optimization capability demonstrated here opens the possibility of evaluating objective function sensitivities with respect to the elements of the design brief in a timely manner. Consider, for example, the impact of the required service life of the
- 溫馨提示:
1: 本站所有資源如無特殊說明,都需要本地電腦安裝OFFICE2007和PDF閱讀器。圖紙軟件為CAD,CAXA,PROE,UG,SolidWorks等.壓縮文件請下載最新的WinRAR軟件解壓。
2: 本站的文檔不包含任何第三方提供的附件圖紙等,如果需要附件,請聯(lián)系上傳者。文件的所有權(quán)益歸上傳用戶所有。
3.本站RAR壓縮包中若帶圖紙,網(wǎng)頁內(nèi)容里面會有圖紙預(yù)覽,若沒有圖紙預(yù)覽就沒有圖紙。
4. 未經(jīng)權(quán)益所有人同意不得將文件中的內(nèi)容挪作商業(yè)或盈利用途。
5. 裝配圖網(wǎng)僅提供信息存儲空間,僅對用戶上傳內(nèi)容的表現(xiàn)方式做保護處理,對用戶上傳分享的文檔內(nèi)容本身不做任何修改或編輯,并不能對任何下載內(nèi)容負責(zé)。
6. 下載文件中如有侵權(quán)或不適當內(nèi)容,請與我們聯(lián)系,我們立即糾正。
7. 本站不保證下載資源的準確性、安全性和完整性, 同時也不承擔(dān)用戶因使用這些下載資源對自己和他人造成任何形式的傷害或損失。
最新文檔
- 企業(yè)生產(chǎn)決策報告
- 進口鐵礦粉的燒結(jié)性能及配礦方法
- 經(jīng)濟學(xué)說史第十四章新凱恩斯主義
- 時間管理從拖延走向高效的基石
- (聽賞)月光下的鳳尾竹
- 課題1水的組成 (10)(精品)
- 客戶溝通方法與技巧
- 大中華國際交易廣場寫字樓項目營銷推廣報告
- 易拉罐尺寸的最優(yōu)設(shè)計方案
- 智慧教室核舟記
- 信息化 BI 商業(yè)智能與企業(yè)即時戰(zhàn)情中心
- 語文蘇教版六年級上冊《船長》第一課時
- 曲軸工藝基礎(chǔ)知識
- 電信集團網(wǎng)規(guī)網(wǎng)優(yōu)A+級培訓(xùn)——11-CDMA功率控制及參數(shù)設(shè)置
- 三、物質(zhì)的密度 (2)